“Is anyone using this?” – Usage Tracking is Timeless
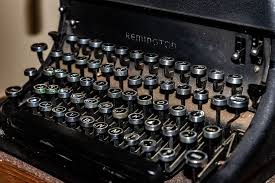
Even before mainframe computing became an enterprise standard, system managers had to monitor resource usage to control costs, govern processing loads and make decisions about system capacity. Granted, this topic today seems so 1960’s… only to be discussed in the smoking lounge at NASA, but these concerns still exist in both mainframes and cloud computing environments.
Today the concerns have shifted somewhat from processing costs to processing value. Thankfully, data-driven organizations can maximize this value by monitoring their information usage patterns on an ongoing basis. In this post, I am revisiting the timeless practice of “usage tracking”, with a focus on analytic solutions. Collecting usage information helps organizations measure the “who”, the “what”, the “when” and “for how long” questions around their reporting and analytics investments.
Why do I still care? This topic resurfaced for me this past year, when a few different clients and colleagues called out the need for better tracking of their analytics usage. A variety of platform-based or home-grown solutions can track your usage, and you can use their metrics at any time. Let’s look at some of the major secrets this practice can reveal.
The “Who”
I will get this aspect out of the way first. Tracking your users in the ‘data security’ context is not what I am discussing here. While that is an important factor, it should be addressed at an enterprise level – much higher in the order of things than data solution usage.
Identifying who your primary analytic users are helps you understand the key audiences using the solutions you maintain. In addition, knowing what analysis those users depend on helps you understand the questions they are asking of your data. Meshing those two bits of knowledge together can power your decision making around future analytics. For example, if the Operations team spends time pulling analysis from both operations and finance sources, it is likely that these users are under-served in the finance scope. The scope of their analytic tools should include the finance data they need.
The “What”
Employing usage tracking helps you learn what analysis is being requested. Having this knowledge can help optimize how you invest your analytics budget. As mentioned above, it is vital to combine the what with the who to better understand usage patterns across on an organization. Moreover, focusing on what analysis is NOT being requested can help you trim back on maintaining analytics nobody uses. Business models and priorities can evolve over time, and some analysis is not as important as it used to be. This may offer opportunities to retire some reports and possibly some of the processing required to produce this analysis.
One challenge to usage tracking involves data portability. Some users export data from the analytic tools in order to combine it with external data – data that might otherwise be added to the what we could then track. If we think about the value that we expect from our data solutions, it makes sense to include this external data in our collection so we can save users time.
The “When”
Do your users only request certain analysis at certain times of the year? Do they only run reports in the mornings? Unless there are major processing constraints, I prefer making ALL data available ALL the time. Usage tracking can be used to pinpoint times of peak usage, but when is this helpful? I will ask readers to weigh in on this one (please leave a comment), as I am sure there are other reasons besides these:
- If your analytics usage is cloud-based and limited to certain hours of the day, you can optimize cloud resource utilization and cost with a defined schedule. Usage tracking will aid in your scheduling decisions, but the schedule may need to be modified during seasonal spikes.
- Maintenance and processing constraints need to be coordinated with usage periods. Usage tracking can help define the “window” with which data availability should be guaranteed. Processing time, scheduled maintenance, and other administrative activities can then be coordinated outside of that timeframe window.
- What else? (Please comment below)
“For how long?”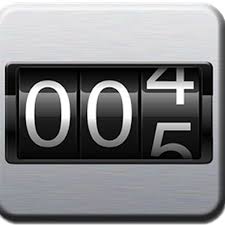
With some usage tracking, we can measure how long the analytic queries run. We can also measure the run duration of batch-processed reports (which are still around). This gives administrators insight into performance issues and opportunities for optimizing. If performance suffers, so does the user’s perception of the solution itself.
Another aspect to measure is how often and how long the users spend each week running their analysis. Users assembling data each week or month by running the same set of reports is a red flag, especially if there is manual effort being done each time to assemble the analysis. This is an opportunity to automate the queries and assembly work into a finished product report or analysis package. If this usage pattern is missed during the requirements phase, usage tracking can reveal those opportunities.
The Blind Spot
One last mention. Even with persistent monitoring of usage activity, we lack visibility into where else our data solutions need to expand. External data analyzed by your users might be data of value as mentioned previously, and it is an example of where the scope of analytics needs to expand. This is a known reality with data scientists, who have historically been tasked with data collection and prep work that may not be necessary if it can be collected upstream.
Usage statistics will show a trend of what information gets used by whom, but it does not replace the need to talk to users about other questions they need answered from the data. That type of polling requires a more interactive approach.
Thanks, this site is very practical.